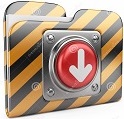
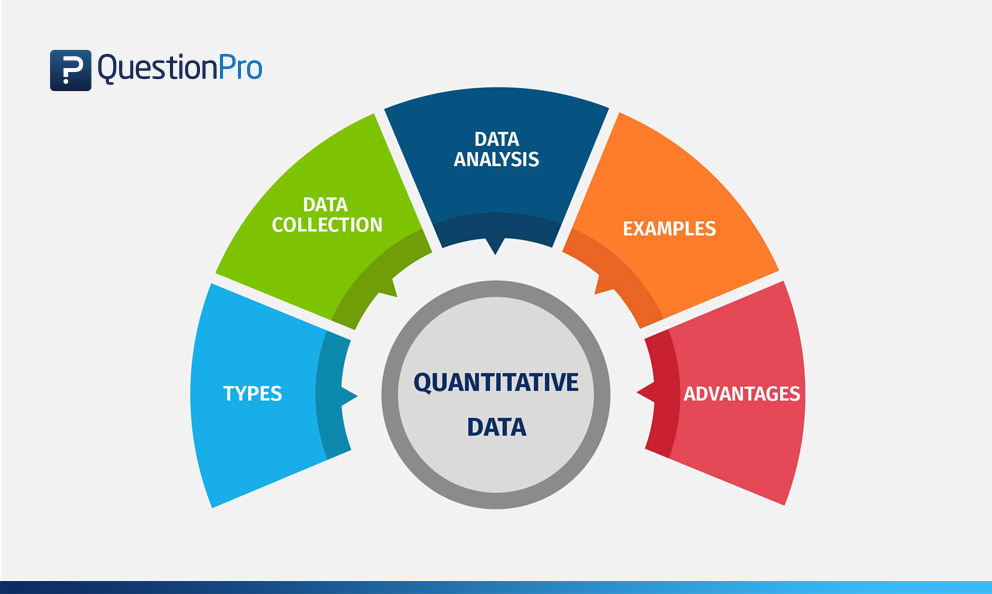
Depending on the method, the number of observations within subjects can vary across subjects, and the time intervals between observations can vary within as well as between subjects (in examples from our longitudinal studies, observations are often months to >1 year apart). By ‘longitudinal research’, we generally restrict ourselves here to data sets where typically each of a moderate/large number of subjects (10 to hundreds) has a relatively small number of repeat readings on a single, continuous, interval-level, numeric measure across time, usually 2–30 observations per subject over time. In our discussions, we focus more on longitudinal observational research (prospective and retrospective), and to a lesser extent on randomized interventions or randomized clinical trials.
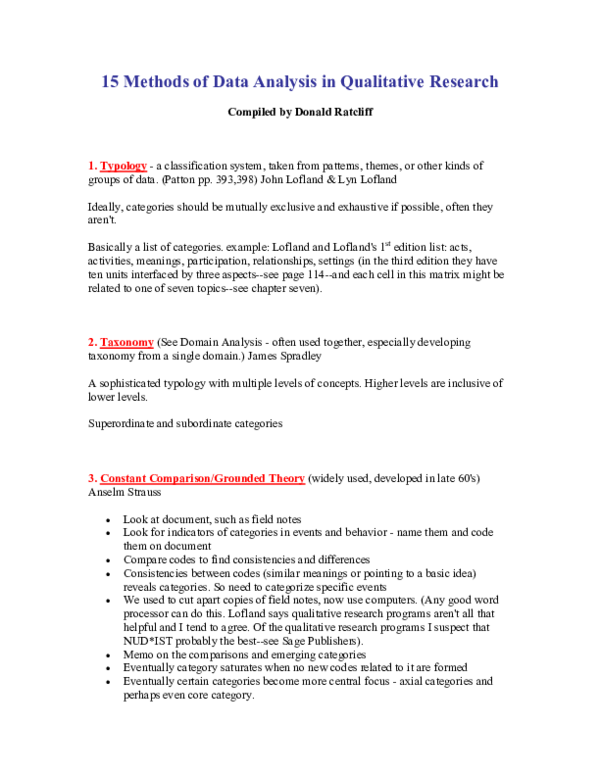
#Data analysis methods examples software
For readers wanting user-friendly drop-down menus, SPSS and JMP software provide analysis options with some of the advanced modeling techniques reviewed here (e.g., repeated-measure analysis of covariance, ANCOVA, and mixed-effect models). To be accessible to a wide audience, our format leans toward verbal, intuitive, and graphical presentation with examples, software suggestions, and programming code/scripts in SAS and MPlus software. While we assume familiarity with basic methods of descriptive and inferential statistics for the biological, medical, and/or behavioral sciences (e.g., analysis of variance, ANOVA, and regression/correlation), our approach does not require specialized or advanced knowledge of statistics or modeling. In this review, we provide, using an informal and straightforward style, an organized overview of the types of methods available and suggest approaches for situations under which they may be appropriate. While we emphasize that sophisticated and advanced analytic models cannot, and should not, compensate for poor study design and execution, we also maintain that solely using simplistic analytic methods can scuttle detection of important signals and effects, even in well-designed and -conducted studies. This lack of understanding can lead to inappropriate or inefficient analysis, inaccurate results, and simplistic or wrong interpretations, conclusions, and judgments. We feel there is too often a general lack of understanding and confusion concerning appropriate longitudinal data analysis methods that has bred insecurity towards or prejudice against the use of newer, advanced and more powerful methods among some clinical researchers and journal reviewers of neurological literature. Substantively, the special focus is on neurological conditions, especially dementia, but the methods are more broadly relevant. This paper provides a broad didactic survey of methods for statistical analysis of longitudinal, clinical, observational and experimental data, illustrated by applied examples, aimed to be of practical utility for clinical researchers with little background in statistical modeling.
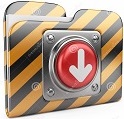